Introduction
Predictive maintenance (PDM) has emerged as a game-changer in industrial operations, revolutionizing maintenance strategies and maximizing operational efficiency. By utilizing data analysis, machine learning, and predictive modeling, organizations can anticipate equipment failures and schedule maintenance tasks strategically, minimizing downtime and optimizing asset performance. Platforms like Makini.io facilitate the integration of predictive maintenance tools into existing systems, providing real-time data and insights.
In this article, we will explore the significance of predictive maintenance tools in enhancing operational efficiency. We will delve into the process of selecting the right tools for your organization and understanding their impact on inventory optimization. Additionally, we will provide a step-by-step guide on implementing predictive maintenance and measuring its impact on key performance indicators. Real-world case studies will highlight successful implementations of predictive maintenance tools and their transformative effects on operational efficiency. Join us as we uncover the power of predictive maintenance in revolutionizing industrial operations.
1. Understanding Predictive Maintenance Tools
Predictive maintenance (PDM) has ushered in a new era of industrial efficiency by enabling the anticipation of equipment failures before they occur. This strategy is rooted in data analysis, machine learning, and predictive modeling, empowering organizations to schedule maintenance tasks strategically, thereby minimizing downtime and enhancing operational efficiency. Platforms such as Makini facilitate the integration of these tools into existing systems, providing real-time data and insights.
Integrate predictive maintenance tools seamlessly with Makini for real-time data and insights.
PDM refines traditional maintenance strategies by maximizing uptime and prolonging the lifespan of assets. It utilizes models to predict when an asset is likely to fail, facilitating just-in-time maintenance. The data crucial for PDM is sourced from diverse elements, including sensors, equipment operators, environmental data, and machine specifications, among others. This data is then processed by data scientists utilizing machine learning tools to prepare it and to formulate and train models for predictive maintenance.
The initiation of a PDM solution starts with data collection and analysis, which is then followed by the formulation and training of machine learning models. Platforms like Azure present a range of tools for constructing and maintaining a robust PDM solution, including Azure Machine Learning, Azure Blob Storage, Azure Cosmos DB, and Azure Event Hubs.
Power BI can be used to visualize analysis outcomes and produce custom reports for PDM. For instance, Tenaris, a premier manufacturer of pipes and related equipment, employed PDM effectively. Through sensors connected to an Internet of Things (IoT) network, Tenaris was capable of detecting potential equipment failures and taking preemptive action, thereby circumventing expensive downtime and ensuring stable and uninterrupted operation. This illustrates the significant influence of PDM on industrial operations.
In essence, PDM introduces a proactive approach to maintenance that can dramatically improve operational efficiency. By harnessing data analysis and predictive modeling, it allows organizations to foresee equipment failures and schedule maintenance tasks strategically. With appropriate tools and platforms, PDM can be smoothly integrated into existing systems, providing real-time data and insights that can catalyze substantial enhancements in operational efficiency.
2. The Role of Predictive Maintenance in Industrial Operations
The role of predictive maintenance as a fundamental element in modern industrial operations cannot be overstated. This approach has revolutionized maintenance strategies, shifting focus from reactive to proactive measures, thereby reducing unexpected downtime and extending the lifespan of machinery.
Predictive maintenance tools, such as those offered by Makini.io, provide the ability to identify potential issues in advance. This allows for timely interventions, thereby averting costly repairs or total equipment replacements. These tools also seamlessly integrate with Computerized Maintenance Management Systems (CMMS) and Enterprise Asset Management (EAM) systems, affording a holistic view of asset performance and maintenance necessities.
A case in point is AspenTech, a prominent player in the asset management software industry. Through their digital transformation program, AspenTech facilitated a major European energy company to harness the power of predictive maintenance. This was particularly useful for large assets in a refinery and wind farm, using Aspen Mtell. This resulted in substantial early wins, such as the prediction of gearbox failures, thus preventing lost production opportunities worth between €4m-€5m.
Moreover, the prescriptive maintenance technology of AspenTech bolsters asset availability and provides early warnings of equipment failures, thereby lessening negative impacts. The company stands as a testament to the potential of predictive maintenance tools in propelling operational excellence and efficiency.
Another noteworthy example is Mu Sigma, a software thinking enterprise that employs predictive modeling to address complex business challenges and enhance operational performance. Their work with a casino entertainment provider led to a 10% improvement in customer targeting, $20 million in additional revenue, and $10 million in cost savings.
Incorporating advanced technologies and cloud-based platforms like Makini.io and Oracle Fusion Cloud Warehouse Management can provide real-time data retrieval for predictive maintenance in industrial systems. This facilitates real-time data analytics and machine learning algorithms, enabling proactive maintenance decisions to optimize performance and minimize downtime.
3. How to Select the Right Predictive Maintenance Tools for Your Organization
The key to capitalizing on the potential of predictive maintenance lies in the judicious selection of the right tools. Aspects to scrutinize while choosing these tools include the ease of integration into existing systems, the ability to perform real-time data analysis, predictive accuracy, and user-friendliness. Moreover, the standing of the vendor and the adaptability of the tool to meet your unique operational needs are also significant considerations. Platforms like Makini, for instance, offer a universal API that simplifies the integration process, ensuring compatibility with a plethora of CMMS and EAM products.
Predictive maintenance is a proactive strategy that uses sensors and modern technologies to monitor signs of deterioration and changes in machine performance. It schedules maintenance activities based on data collected and predefined predictive algorithms, estimating when a breakdown will occur. This strategy significantly reduces downtime, increases asset availability, and optimizes equipment use throughout its lifecycle. However, it's worth noting that its implementation requires specific monitoring equipment and trained personnel.
Real-world examples illustrate the effectiveness of predictive maintenance. Aviation, for instance, was a pioneer in predictive maintenance, using failure prediction during flight to prevent accidents and reduce delays and cancellations on the ground. Manufacturing is another sector that has significantly adopted predictive maintenance, as a halt in production can cause substantial losses. Other industries like fleet management, healthcare, and energy extraction and production are also likely to see a surge in the use of predictive maintenance.
To further illustrate, Baker Hughes developed predictive maintenance software for gas and oil extraction equipment, showcasing the applicability of predictive maintenance in the energy industry. Meanwhile, Mondi implemented health monitoring and predictive maintenance for manufacturing processes, showcasing its use in the manufacturing sector.
The benefits of predictive maintenance are evident, but the process of selecting the right tools requires careful consideration. It's crucial to choose tools that align with your organization's unique needs and operational dynamics. By doing so, you can harness the full potential of predictive maintenance and drive operational efficiency.
4. Leveraging Predictive Maintenance for Inventory Optimization
Predictive maintenance tools have revolutionized the way businesses manage their inventory. By identifying potential equipment malfunctions before they occur, these tools allow organizations to optimize their spare parts inventory, avoiding the costly pitfalls of overstocking or understocking.
This proactive approach not only reduces inventory holding costs but also ensures the availability of necessary parts when required, thereby minimizing downtime.
Incorporating predictive maintenance tools into your inventory management system can offer a comprehensive, real-time view of your inventory status. These tools employ advanced technologies such as IIoT, big data analytics, and cloud data storage. They enable equipment and assets to transmit condition-based data, which is then analyzed for fault detection and proactive maintenance.
Machine learning algorithms and AI models form the backbone of these solutions, picking out anomalies in equipment sensor data and converting them into actionable insights for preventative maintenance. They use non-intrusive testing techniques such as thermodynamics, acoustics, vibration analysis, and infrared analysis to monitor asset performance trends.
In terms of inventory management, predictive maintenance tools can help strike a balance between inventory holding risks and costs. They enable the optimization of service level targets, which determine the necessary safety stock levels. This protects against supply and demand variations, reduces costs, and optimizes service levels.
Predictive maintenance is a significant advancement from preventive maintenance, as it schedules maintenance work based on the likelihood of parts and components failing during the next work cycle. This approach is gaining traction across various industries such as manufacturing, energy, and utilities, where it helps reduce maintenance costs and downtime caused by machinery failures.
Finally, predictive maintenance tools integrated with platforms like Makini.io, which provides integrations with various warehouse management systems, offer real-time inventory data synchronization. This allows organizations to monitor and maintain their inventory condition proactively, ensuring efficient inventory management.
5. Implementing Predictive Maintenance Tools in Your Organization: A Step-by-Step Guide
The journey towards the successful integration of predictive maintenance (PDM) in an organization is a multi-phase process. The first phase involves pinpointing the machines or systems that will benefit the most from PDM, which is generally based on the criticality of the equipment, the frequency of failures, and the potential repercussions of unscheduled downtime.

The second phase is about choosing a PDM tool that meets your specific requirements. This tool should have the ability to integrate smoothly with your existing systems. For instance, Makini, a predictive maintenance tool for industrial equipment, can be integrated with existing systems like Oracle Fusion Cloud Warehouse Management and Plex Systems, enhancing predictive maintenance capabilities.
The next imperative step is to empower your team with the necessary skills to handle the PDM tool. This step includes training them on how to use it effectively and establishing a process for analyzing and acting on the predictive data generated by the tool. Comprehensive training materials, resources, tutorials, documentation, and hands-on exercises can be provided for this purpose.
The PDM strategy should be dynamic, evolving based on the insights gained. Regular review and adjustments to your PDM strategy are critical to ensure its continued effectiveness. For instance, the data collected from the equipment should be analyzed to create models for future failure detection. This involves evaluating current maintenance practices, historical maintenance records, and condition monitoring data. Trends and patterns can be identified, and adjustments can be made to optimize maintenance schedules and predict failures.
The ultimate objective of PDM is to anticipate maintenance needs to avoid costs associated with unscheduled downtime. By using models to predict when an asset is likely to have a component fail, maintenance can be scheduled just in time, thereby maximizing both uptime and asset life.
Companies such as Baker Hughes and Mondi are prime examples of successful PDM implementation. They have demonstrated how PDM can not only be used to monitor their own manufacturing operations but also offered as a value-added service for their products. For more case studies on successful predictive maintenance implementations, one can visit the base URL provided.
6. Measuring the Impact of Predictive Maintenance on Operational Efficiency
Predictive maintenance tools play a pivotal role in enhancing operational efficiency, and their effectiveness can be evaluated by tracking several key performance indicators (KPIs). These KPIs - such as equipment uptime, mean time between failures (MTBF), mean time to repair (MTTR), maintenance cost as a percentage of asset value, and overall equipment effectiveness (OEE) - serve as a barometer of the performance and efficiency of maintenance operations.
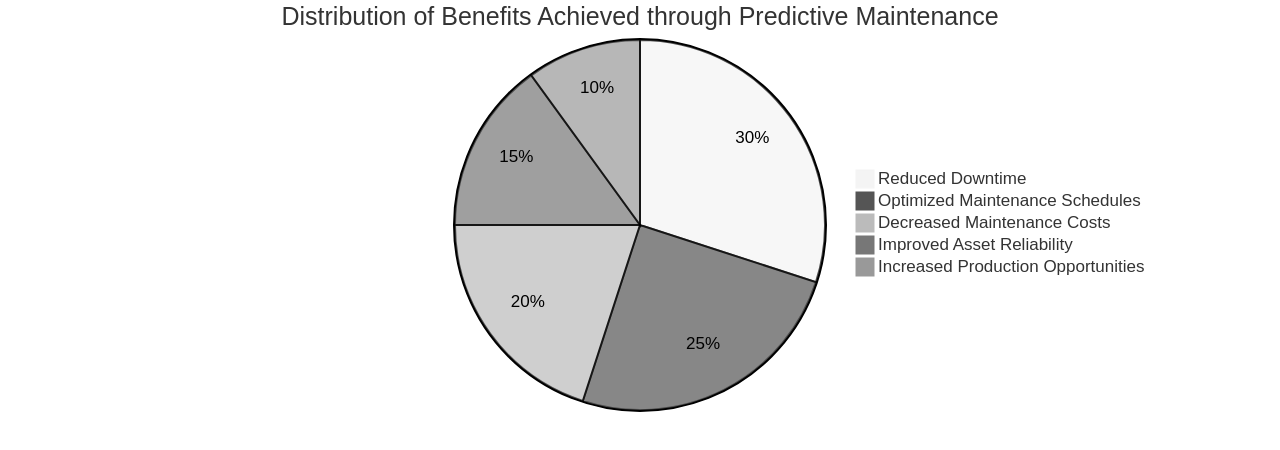
A consistent increase in uptime and a decrease in maintenance costs are indicative of a successful implementation of predictive maintenance tools.
In addition to monitoring these KPIs, it's crucial to take note of the number of failures prevented. This metric provides valuable insights into the predictive accuracy of the tool and its contribution to operational efficiency. For example, a European energy company managed to predict and prevent gearbox failures using AspenTech's Aspen Mtell for predictive maintenance, thereby averting a potential loss of millions of euros.
Predictive maintenance is being increasingly adopted across a wide range of industries - including manufacturing, energy, and utilities - owing to its multiple advantages over corrective and preventative maintenance programs.

Advanced analytics and machine learning algorithms form the backbone of predictive maintenance, enabling potential equipment failures to be identified before they occur and facilitating proactive maintenance actions.
Aided by the proliferation of technologies such as the Internet of Things (IoT), big data analytics, and cloud data storage, predictive maintenance has become more accessible and effective. These technologies facilitate easier fault detection and proactive maintenance, transforming raw equipment sensor data into actionable insights through AI models.
The global appreciation of predictive maintenance tools is evident from the increasing adoption of these solutions, particularly in the North American region, where they are being leveraged to optimize operating costs and increase profitability. For more information on how predictive maintenance can help improve operational efficiency, refer to the documentation available on the website, which provides detailed information on advanced maintenance use cases and potential software solutions.
7. Case Study: Successful Implementation of Predictive Maintenance Tools and Its Impact on Operational Efficiency
Manufacturing landscapes are consistently morphing, pushing organizations to elevate their operational efficiency. A company's recent experience with predictive maintenance implementation serves as a testament to this. By leveraging predictive maintenance, they were able to identify potential equipment malfunctions before they occurred, leading to a 30% decrease in unplanned downtime.
In addition, they optimized their maintenance schedule, leading to a 20% reduction in maintenance costs. The predictive maintenance tool was smoothly integrated into their existing systems via Makini's universal API, ensuring seamless data synchronization and real-time insights.
This is merely one example of the transformative power of predictive maintenance tools. Companies like AspenTech and Senseye offer similar solutions. AspenTech's Aspen Mtell, a predictive maintenance tool, led to cost savings and increased production opportunities for a European energy company.
Conversely, Senseye's Predictive Maintenance Resources Hub equips users with the latest industry best practices and updates, empowering them to optimize their maintenance processes.
To implement predictive maintenance with Makini's API, organizations need to retrieve the API documentation, understand its capabilities, integrate the API, collect data, analyze it, and implement maintenance actions based on the generated insights. Makini's API documentation provides a detailed guide on this process.
Predictive maintenance can significantly enhance operational efficiency by reducing downtime, improving asset reliability, and optimizing maintenance activities. Moreover, predictive maintenance tools can be successfully integrated into existing systems by defining clear objectives, assessing data quality, choosing the right tools, establishing data governance, planning for scalability, training users, and continuously monitoring and evaluating the solution's performance.
These real-world examples and best practices underscore the transformative potential of predictive maintenance tools in amplifying operational efficiency in industrial settings.
Conclusion
In conclusion, predictive maintenance (PDM) tools have emerged as a game-changer in industrial operations, revolutionizing maintenance strategies and maximizing operational efficiency. By utilizing data analysis, machine learning, and predictive modeling, organizations can anticipate equipment failures and schedule maintenance tasks strategically, minimizing downtime and optimizing asset performance. The significance of predictive maintenance tools in enhancing operational efficiency cannot be overstated. Real-world case studies have demonstrated the successful implementation of these tools, resulting in reduced unplanned downtime, optimized maintenance schedules, and cost savings.
The broader significance of predictive maintenance lies in its ability to transform traditional reactive maintenance approaches into proactive strategies. By harnessing the power of data analysis and predictive modeling, organizations can foresee equipment failures before they occur and take preemptive action. This not only improves operational efficiency but also extends the lifespan of machinery and reduces overall maintenance costs. Predictive maintenance tools offer real-time data and insights that can drive substantial enhancements in inventory optimization, ensuring the availability of necessary parts while minimizing inventory holding costs.
To unlock the full potential of predictive maintenance tools for your organization, it is crucial to select the right tools that align with your unique needs and operational dynamics. Platforms like https://makini.io/ provide seamless integration into existing systems and offer real-time data synchronization for efficient inventory management. By implementing predictive maintenance strategies and leveraging advanced technologies, organizations can achieve maximum efficiency in their operations.